

-
需要太多标签化的训练数据。
-
模型的训练时间太长或者需要太多昂贵的资源,而且还可能根本无法训练。
-
超参数,尤其是围绕节点和层的超参数,仍然是神秘的。自动化甚至是公认的经验法则仍然遥不可及。
-
迁移学习,意味着只能从复杂到简单,而不是从一个逻辑系统到另一个逻辑系统。


Capsule是一组嵌套的神经层。在普通的神经网络中,你会不断地添加更多的层。在CapsNet中,你会在一个单层内增加更多的层。或者换句话说,把一个神经层嵌套在另一个神经层里面。capsule里面的神经元的状态就能捕捉到图像里面一个实体的上述属性。一个胶囊输出一个向量来代表实体的存在。向量的方向代表实体的属性。该向量被发送到神经网络中所有可能的父代。预测向量是基于自身权重和权重矩阵相乘计算的。哪个父代的标量预测向量乘积最大,哪个父代就会增加胶囊的结合度。其余的父代则降低其结合度。这种通过协议的路由方式优于目前的max-pooling等机制。
-
只需要训练数据的一小部分。 -
在您的桌面CPU设备上运行,无需GPU。 -
训练速度一样快,在许多情况下甚至更快,适合分布式处理。 -
超参数少得多,在默认设置下表现良好。 -
依靠容易理解的随机森林,而不是完全不透明的深度神经网。


<pre style="max-width: 100%;box-sizing: border-box !important;overflow-wrap: break-word !important;"><section style="max-width: 100%;letter-spacing: 0.544px;white-space: normal;font-family: -apple-system-font, system-ui, "Helvetica Neue", "PingFang SC", "Hiragino Sans GB", "Microsoft YaHei UI", "Microsoft YaHei", Arial, sans-serif;box-sizing: border-box !important;overflow-wrap: break-word !important;"><section powered-by="xiumi.us" style="max-width: 100%;box-sizing: border-box !important;overflow-wrap: break-word !important;"><section style="margin-top: 15px;margin-bottom: 25px;max-width: 100%;opacity: 0.8;box-sizing: border-box !important;overflow-wrap: break-word !important;"><section style="max-width: 100%;box-sizing: border-box !important;overflow-wrap: break-word !important;"><section style="max-width: 100%;letter-spacing: 0.544px;box-sizing: border-box !important;overflow-wrap: break-word !important;"><section powered-by="xiumi.us" style="max-width: 100%;box-sizing: border-box !important;overflow-wrap: break-word !important;"><section style="margin-top: 15px;margin-bottom: 25px;max-width: 100%;opacity: 0.8;box-sizing: border-box !important;overflow-wrap: break-word !important;"><section><section style="margin-bottom: 15px;padding-right: 0em;padding-left: 0em;max-width: 100%;color: rgb(127, 127, 127);font-size: 12px;font-family: sans-serif;line-height: 25.5938px;letter-spacing: 3px;box-sizing: border-box !important;overflow-wrap: break-word !important;"><span style="max-width: 100%;color: rgb(0, 0, 0);box-sizing: border-box !important;overflow-wrap: break-word !important;"><strong style="max-width: 100%;box-sizing: border-box !important;overflow-wrap: break-word !important;"><span style="max-width: 100%;font-size: 16px;font-family: 微软雅黑;caret-color: red;box-sizing: border-box !important;overflow-wrap: break-word !important;">为您推荐</span></strong></span></section><section style="margin-top: 5px;margin-bottom: 5px;padding-right: 0em;padding-left: 0em;max-width: 100%;min-height: 1em;font-family: sans-serif;letter-spacing: 0px;opacity: 0.8;line-height: normal;box-sizing: border-box !important;overflow-wrap: break-word !important;">干货 | 算法工程师超实用技术路线图</section><section style="margin-top: 5px;margin-bottom: 5px;padding-right: 0em;padding-left: 0em;max-width: 100%;min-height: 1em;font-family: sans-serif;letter-spacing: 0px;opacity: 0.8;line-height: normal;box-sizing: border-box !important;overflow-wrap: break-word !important;"><span style="font-size: 14px;">那些轻轻拍了拍Attention的后浪们</span></section><section style="margin-top: 5px;margin-bottom: 5px;padding-right: 0em;padding-left: 0em;max-width: 100%;min-height: 1em;font-family: sans-serif;letter-spacing: 0px;opacity: 0.8;line-height: normal;box-sizing: border-box !important;overflow-wrap: break-word !important;">吴恩达推荐笔记:22 张图总结深度学习全部知识</section><section style="margin-top: 5px;margin-bottom: 5px;padding-right: 0em;padding-left: 0em;max-width: 100%;min-height: 1em;font-family: sans-serif;letter-spacing: 0px;opacity: 0.8;line-height: normal;color: rgb(0, 0, 0);box-sizing: border-box !important;overflow-wrap: break-word !important;"><span style="font-size: 14px;">周博磊自述:一个神经元的价值和一个神经病的坚持</span></section><section style="margin-top: 5px;margin-bottom: 5px;padding-right: 0em;padding-left: 0em;max-width: 100%;min-height: 1em;font-family: sans-serif;letter-spacing: 0px;opacity: 0.8;line-height: normal;color: rgb(0, 0, 0);box-sizing: border-box !important;overflow-wrap: break-word !important;">你一定从未看过如此通俗易懂的YOLO系列解读 (下)</section></section></section></section></section></section></section></section></section>
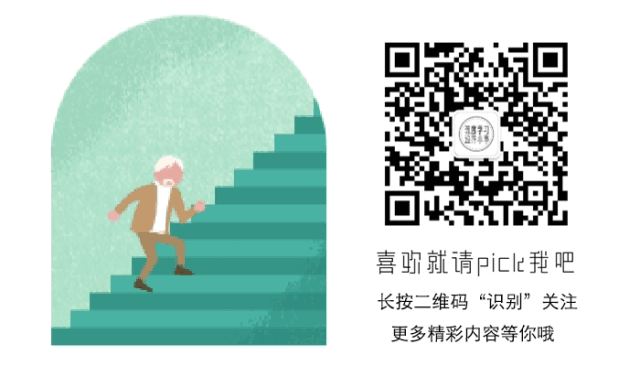
本篇文章来源于: 深度学习这件小事
本文为原创文章,版权归知行编程网所有,欢迎分享本文,转载请保留出处!
内容反馈