来源 | towardsdatascience
【导读】近日,两位Facebook的研究员小哥,提出了一种新的对比各种自监督学习框架的方法,结果显示SimCLR、CPC、AMDIM这些框架,彼此之间并无本质差异。
对比自监督学习将取代监督式深度学习主导地位的预言,已经「甚嚣尘上」。
Hinton大力推广的SimCLR就是其中一种。
对比自监督学习(CLS)是从无标签数据中选择和对比Anchor,Positive,Negative(APN)三种特征,学习到有用表征的方法。
近日,Facebook的两位研究员,使用一个新的框架来对比分析了目前三个主流的自监督学习算法:SimCLR、CPC、AMDIM。结果显示,尽管这些方法表面上看起来非常不同,但实际上他们互相之间只有微小的差异。
回顾:自监督学习和对比学习

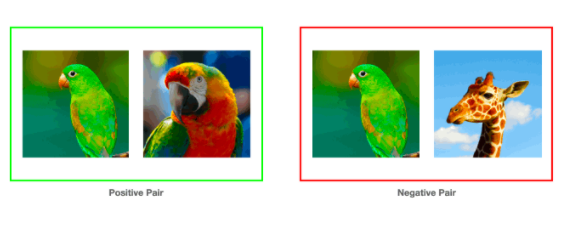

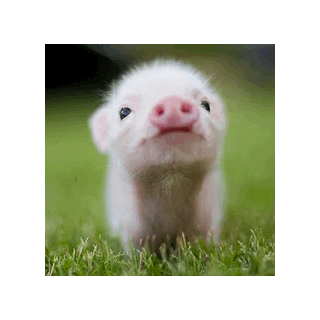
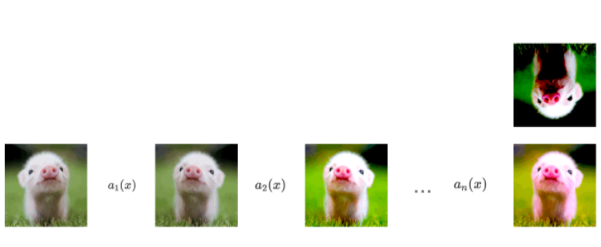


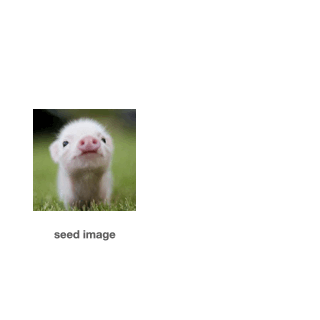


<section style="white-space: normal;line-height: 1.75em;text-align: center;"><strong style="color: rgb(0, 0, 0);font-family: -apple-system-font, system-ui, "Helvetica Neue", "PingFang SC", "Hiragino Sans GB", "Microsoft YaHei UI", "Microsoft YaHei", Arial, sans-serif;letter-spacing: 0.544px;widows: 1;background-color: rgb(255, 255, 255);max-width: 100%;box-sizing: border-box !important;overflow-wrap: break-word !important;"><span style="max-width: 100%;letter-spacing: 0.5px;font-size: 14px;box-sizing: border-box !important;overflow-wrap: break-word !important;"><strong style="max-width: 100%;font-size: 16px;letter-spacing: 0.544px;box-sizing: border-box !important;overflow-wrap: break-word !important;"><span style="max-width: 100%;letter-spacing: 0.5px;box-sizing: border-box !important;overflow-wrap: break-word !important;">—</span></strong>完<strong style="max-width: 100%;font-size: 16px;letter-spacing: 0.544px;box-sizing: border-box !important;overflow-wrap: break-word !important;"><span style="max-width: 100%;letter-spacing: 0.5px;font-size: 14px;box-sizing: border-box !important;overflow-wrap: break-word !important;"><strong style="max-width: 100%;font-size: 16px;letter-spacing: 0.544px;box-sizing: border-box !important;overflow-wrap: break-word !important;"><span style="max-width: 100%;letter-spacing: 0.5px;box-sizing: border-box !important;overflow-wrap: break-word !important;">—</span></strong></span></strong></span></strong></section><pre><pre style="max-width: 100%;box-sizing: border-box !important;overflow-wrap: break-word !important;"><section style="max-width: 100%;letter-spacing: 0.544px;white-space: normal;font-family: -apple-system-font, system-ui, "Helvetica Neue", "PingFang SC", "Hiragino Sans GB", "Microsoft YaHei UI", "Microsoft YaHei", Arial, sans-serif;widows: 1;box-sizing: border-box !important;overflow-wrap: break-word !important;"><section powered-by="xiumi.us" style="max-width: 100%;box-sizing: border-box !important;overflow-wrap: break-word !important;"><section style="margin-top: 15px;margin-bottom: 25px;max-width: 100%;opacity: 0.8;box-sizing: border-box !important;overflow-wrap: break-word !important;"><section style="max-width: 100%;box-sizing: border-box !important;overflow-wrap: break-word !important;"><section style="max-width: 100%;letter-spacing: 0.544px;box-sizing: border-box !important;overflow-wrap: break-word !important;"><section powered-by="xiumi.us" style="max-width: 100%;box-sizing: border-box !important;overflow-wrap: break-word !important;"><section style="margin-top: 15px;margin-bottom: 25px;max-width: 100%;opacity: 0.8;box-sizing: border-box !important;overflow-wrap: break-word !important;"><section><section style="margin-bottom: 15px;padding-right: 0em;padding-left: 0em;max-width: 100%;color: rgb(127, 127, 127);font-size: 12px;font-family: sans-serif;line-height: 25.5938px;letter-spacing: 3px;text-align: center;box-sizing: border-box !important;overflow-wrap: break-word !important;"><span style="max-width: 100%;color: rgb(0, 0, 0);box-sizing: border-box !important;overflow-wrap: break-word !important;"><strong style="max-width: 100%;box-sizing: border-box !important;overflow-wrap: break-word !important;"><span style="max-width: 100%;font-size: 16px;font-family: 微软雅黑;caret-color: red;box-sizing: border-box !important;overflow-wrap: break-word !important;">为您推荐</span></strong></span></section><section style="margin-top: 5px;margin-bottom: 5px;padding-right: 0em;padding-left: 0em;max-width: 100%;min-height: 1em;font-family: sans-serif;letter-spacing: 0px;opacity: 0.8;line-height: normal;text-align: center;box-sizing: border-box !important;overflow-wrap: break-word !important;">那些轻轻拍了拍Attention的后浪们</section><section style="margin-top: 5px;margin-bottom: 5px;padding-right: 0em;padding-left: 0em;max-width: 100%;min-height: 1em;font-family: sans-serif;letter-spacing: 0px;opacity: 0.8;line-height: normal;text-align: center;box-sizing: border-box !important;overflow-wrap: break-word !important;">吴恩达推荐笔记:22 张图总结深度学习全部知识</section><section style="margin-top: 5px;margin-bottom: 5px;padding-right: 0em;padding-left: 0em;max-width: 100%;min-height: 1em;font-family: sans-serif;letter-spacing: 0px;opacity: 0.8;line-height: normal;text-align: center;color: rgb(0, 0, 0);box-sizing: border-box !important;overflow-wrap: break-word !important;">图灵奖得主Yann LeCun《深度学习》春季课程</section><section style="margin-top: 5px;margin-bottom: 5px;padding-right: 0em;padding-left: 0em;max-width: 100%;min-height: 1em;font-family: sans-serif;letter-spacing: 0px;opacity: 0.8;line-height: normal;text-align: center;color: rgb(0, 0, 0);box-sizing: border-box !important;overflow-wrap: break-word !important;">你一定从未看过如此通俗易懂的YOLO系列解读 (下)</section><section style="margin-top: 5px;margin-bottom: 5px;padding-right: 0em;padding-left: 0em;max-width: 100%;min-height: 1em;font-family: sans-serif;letter-spacing: 0px;opacity: 0.8;line-height: normal;text-align: center;color: rgb(0, 0, 0);box-sizing: border-box !important;overflow-wrap: break-word !important;"><span style="color: rgb(87, 107, 149);font-size: 14px;letter-spacing: 0px;">震惊!这个</span>街道办招8人,全是清华北大博士硕士!</section></section></section></section></section></section></section></section></section>
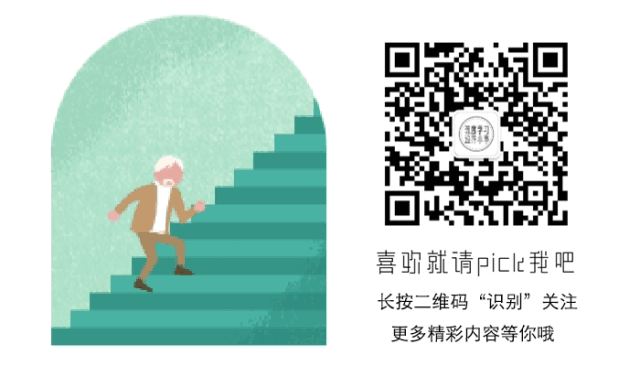
本篇文章来源于: 深度学习这件小事
本文为原创文章,版权归知行编程网所有,欢迎分享本文,转载请保留出处!
内容反馈