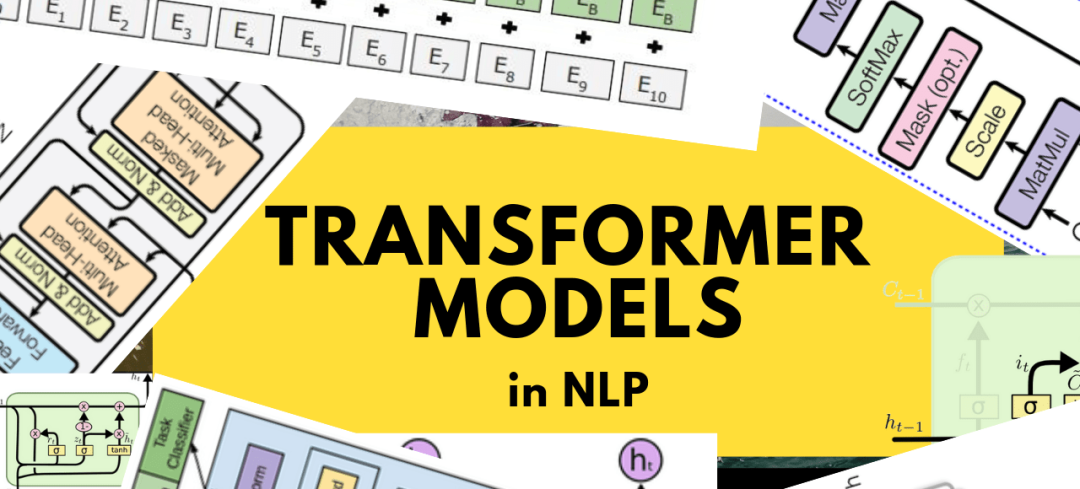
【导读】GitHub超3万星,Transformer更新到第三版,BERT被一分为二,Trainer从类转为方法,还有全新的tokenizer API、TensorFlow改进以及增强的文档和教程。
来了来了,它来了!它带着全新的tokenizer API、TensorFlow改进以及增强的文档和教程来了!
G站上最受欢迎的NLP项目,AI界无人不知的最先进NLP模型Transformer刚刚推出了第三代。
这次更新力度可谓诚意满满,不仅带来了2代的升级,同时还增加了一些新的功能和特性。下面我们就来看看都更新了哪些项目吧。
对比前一代的重大改动
对比前一代的重大改动
首先我们来看看和Transformer v2相比,v3做出了哪些比较令人瞩目的更新。
BERT被一分为二
BERT被分割为BertForMaskedLM和BertLMHeadModel,因此,以后就不能再拿BertForMaskedLM做因果语言建模,也不能接受lm_labels参数。
Trainer从类转为方法
v3还对Trainer数据整理器做了一个改动,将其从一个类改成了一个方法。
直接设置tokenizer的特殊标记属性
在v3中,你可以直接设置tokenizer的特殊标记属性,例如tokenizer.mask_token = '<mask>'。目前v2中,只是将标记关联到tokenizer的属性,但如果该标记不在词汇表中,则不会将其添加到词汇表中,必须使用 tokenizer.add_special_tokens() 和 tokenizer.add_tokens() 方法才能添加标记。
prepare_for_model被移除
作为新的 tokenizer API 的一部分,prepare_for_model 方法被移除。
新的 Tokenizer API
新的 Tokenizer API
随着rust tokenizers的加入,tokenizers在第2版中发展迅速。现在,它有了一个更简单、更灵活的 API,在 Python(slow)和 Rust(fast)tokenizers 之间进行了调整。新的 API 让你可以更深入地控制截断和填充,允许动态填充或填充到 8 的倍数。
重新设计的 API 详细解释:
https://huggingface.co/transformers/master/preprocessing.html。
下面我们来看看这些显著的变化:
-
现在可以截断一个模型的最大输入长度,同时填充一个批次中最长的序列。 -
填充和截断被解耦,更容易控制。 -
它可以pad到预定义长度的倍数例如8,可以为最新的NVIDIA GPU(V100)带来显著的速度提升。 -
一个使用 tokenizer.__call__ 的通用包装器,可用于所有情况(例如单个序列、一对序列到组、批次等)。 -
tokenizer 现在可以接受预标记化的输入。 -
现在,所有的Rust tokenizers都像slow tokenizers一样被全面测试了。 -
一个新类 AddedToken ,用来对添加的标记,在标记化过程中的行为,进行更精细的控制。特别是用户可以控制(1)在标记化过程中,标记周围的左右空格是否会被移除(2)标记是否会在另一个词中被识别,以及(3)标记是否会以标准化的形式被识别(例如,如果标记化器使用小写字母)。 -
序列化问题得到解决 -
在 tokenizers 上使用 return_tensors 参数时,可以创建 NumPy tensors。 -
引入了一个新的枚举 TensorType 来映射我们支持的所有可能的张量后端:TensorType.TENSORFLOW, TensorType.PYTORCH, TensorType.NUMPY。 -
Tokenizers 现在接受 encode(...)、encode_plus(...)、batch_encode_plus(...) tokenizer 方法的 return_tensors 参数的 TensorType enum。 -
BatchEncoding 新增的属性 is_fast 表示 BatchEncoding 是来自 Python(slow)tokenizer 还是 Rust(fast)tokenizer。 -
slow和fast tokenizer 现在是可挑选的。它们的输出也是如此,即 dict 子类 BatchEncoding。
其他激动人心的改进
其他激动人心的改进
针对TensorFlow的改进
全新版本中,针对TensorFlow进行了非常大的升级:
-
TensorFlow模型现在可以自己计算损失,使用TFPretrainedModel.compute_loss方法。 -
现在可以在TensorFlow中调整token嵌入的大小 -
Cleaning TensorFlow model
新增MobileBERT
《MobileBERT: a Compact Task-Agnostic BERT for Resource-Limited Devices 》中的MobileBERT被添加到PyTorch和TensorFlow的库中。
论文地址:
https://arxiv.org/abs/2004.02984
增加了一个单一的检查点:mobilebert-uncased,也就是按照我们的 API 转换的 uncased_L-24_H-128_B-512_A-4_F-4_OPT 检查点。
增强examples/seq2seq内容
全新版本中,将旧的 examples/summarization和 examples/translation文件夹整合到了一个新的examples/seq2seq文件夹中。
-
Finetuning在summarization时效果不错,不过翻译时需要更多的实验。Finetuning可以在多gpu上工作,在验证过程中保存rouge分数,并提供--freeze_encoder和--freeze_embeds选项。这些选项使得BART在cnn/dailymail数据集上的精调速度提高了5倍。 -
在distillation.py中添加了Distillbart代码。目前,它只支持汇总。 -
评估对于summarization和翻译都很好用。 -
新增权重和偏差共享任务,用于XSUM总结任务的协作。
Distilbart
-
Distilbart模型是bart-large-cnn和bart-large-xsum的较小版本,它们可以使用BartForConditionalGeneration.from_pretrained('sshleifer/distilbart-xsum-12-6')来加载 -
重现的命令可以在examples/seq2seq文件夹中找到。
新增BERT Loses Patience
基准
对基准脚本进行了整合,并增加了一些功能。
针对Tensorflow增加推理:CPU、GPU、GPU+XLA、GPU+急切模式、CPU+急切模式、TPU。
针对PyTorch增加了推理和训练:
-
推理:CPU, CPU + torchscript, GPU, GPU + torchscript, GPU + 混合精度, Torch/XLA TPU -
训练:CPU、GPU、GPU+混合精度、Torch/XLA TPU。
更多内容,请移步Transformer的G站页面:
https://github.com/huggingface/transformers/releases/tag/v3.0.0
—完—
<pre style="max-width: 100%;box-sizing: border-box !important;overflow-wrap: break-word !important;"><section style="max-width: 100%;letter-spacing: 0.544px;white-space: normal;font-family: -apple-system-font, system-ui, "Helvetica Neue", "PingFang SC", "Hiragino Sans GB", "Microsoft YaHei UI", "Microsoft YaHei", Arial, sans-serif;widows: 1;box-sizing: border-box !important;overflow-wrap: break-word !important;"><section powered-by="xiumi.us" style="max-width: 100%;box-sizing: border-box !important;overflow-wrap: break-word !important;"><section style="margin-top: 15px;margin-bottom: 25px;max-width: 100%;opacity: 0.8;box-sizing: border-box !important;overflow-wrap: break-word !important;"><section style="max-width: 100%;box-sizing: border-box !important;overflow-wrap: break-word !important;"><section style="max-width: 100%;letter-spacing: 0.544px;box-sizing: border-box !important;overflow-wrap: break-word !important;"><section powered-by="xiumi.us" style="max-width: 100%;box-sizing: border-box !important;overflow-wrap: break-word !important;"><section style="margin-top: 15px;margin-bottom: 25px;max-width: 100%;opacity: 0.8;box-sizing: border-box !important;overflow-wrap: break-word !important;"><section><p style="margin-bottom: 15px;padding-right: 0em;padding-left: 0em;max-width: 100%;color: rgb(127, 127, 127);font-size: 12px;font-family: sans-serif;line-height: 25.5938px;letter-spacing: 3px;text-align: center;box-sizing: border-box !important;overflow-wrap: break-word !important;"><span style="max-width: 100%;color: rgb(0, 0, 0);box-sizing: border-box !important;overflow-wrap: break-word !important;"><strong style="max-width: 100%;box-sizing: border-box !important;overflow-wrap: break-word !important;"><span style="max-width: 100%;font-size: 16px;font-family: 微软雅黑;caret-color: red;box-sizing: border-box !important;overflow-wrap: break-word !important;">为您推荐</span></strong></span></p><p style="margin-top: 5px;margin-bottom: 5px;padding-right: 0em;padding-left: 0em;max-width: 100%;min-height: 1em;font-family: sans-serif;letter-spacing: 0px;opacity: 0.8;line-height: normal;text-align: center;box-sizing: border-box !important;overflow-wrap: break-word !important;">MIT校长评中美科技竞赛:胜利不是期盼对手的失利</p><p style="margin-top: 5px;margin-bottom: 5px;padding-right: 0em;padding-left: 0em;max-width: 100%;min-height: 1em;font-family: sans-serif;letter-spacing: 0px;opacity: 0.8;line-height: normal;text-align: center;box-sizing: border-box !important;overflow-wrap: break-word !important;"><span style="font-size: 14px;">GitHub重大更新:在线开发上线,是时候卸载IDE了</span></p><p style="margin-top: 5px;margin-bottom: 5px;padding-right: 0em;padding-left: 0em;max-width: 100%;min-height: 1em;font-family: sans-serif;letter-spacing: 0px;opacity: 0.8;line-height: normal;text-align: center;box-sizing: border-box !important;overflow-wrap: break-word !important;"><span style="font-size: 14px;">美国官宣117000名 IT 人失业,真是史无前例!</span><br /></p><p style="margin-top: 5px;margin-bottom: 5px;padding-right: 0em;padding-left: 0em;max-width: 100%;min-height: 1em;font-family: sans-serif;letter-spacing: 0px;opacity: 0.8;line-height: normal;text-align: center;box-sizing: border-box !important;overflow-wrap: break-word !important;">数据分析入门常用的23个牛逼Pandas代码</p><section style="margin: 5px 8px;padding-right: 0em;padding-left: 0em;max-width: 100%;min-height: 1em;font-family: sans-serif;letter-spacing: 0px;opacity: 0.8;line-height: normal;text-align: center;box-sizing: border-box !important;overflow-wrap: break-word !important;">特朗普拿H1B签证开刀,LeCun吴恩达等实名谴责!<br /></section></section></section></section></section></section></section></section></section>
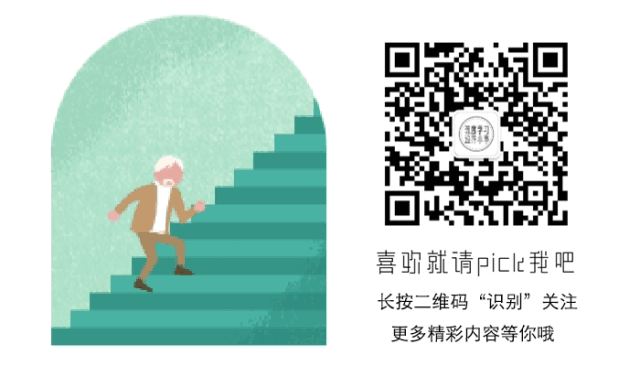
本篇文章来源于: 深度学习这件小事
本文为原创文章,版权归知行编程网所有,欢迎分享本文,转载请保留出处!
你可能也喜欢
- ♥ Longformer:超越RoBERTa,为长文档而生的预训练模型01/20
- ♥ ACL2020结果已出,录用论文抢先看!05/21
- ♥ MIT大神利用半监督or自监督学习,巧妙破解数据不平衡问题!07/03
- ♥ 颜宁追问4位男科学家:如何平衡事业和家庭?07/25
- ♥ “卷积”其实没那么难以理解08/01
- ♥ 图像识别的可视化解释史07/17
内容反馈