选自 | Medium 作者 | Ajinkya Khalwadekar
转自 | 机器之心
前言
在机器学习和计算机视觉领域,光学字符识别(OCR)和手写文本识别(HTR)长期以来都是人们研究的重要主题。本文将帮助计算机视觉爱好者大致了解如何对文档图像中的文本进行识别。
光学字符识别和手写文本识别是人工智能领域里非常经典的问题。OCR 很简单,就是将文档照片或场景照片转换为机器编码的文本;而 HTR 就是对手写文本进行同样的操作。作者在文章中将这个问题分解成了一组更小型的问题,并制作了如下的流程图。
图 1.1:应用流程图
按文档边框裁剪图像
在图像处理中,通常需要对图像进行预先编辑,以便获得更好的表征。裁剪是图像编辑中最常用的操作之一,这可以移除图像中不需要的部分,也可以向图像添加所需的特征。
你可以使用 OpenCV 来轻松地找到图像中文档的边缘,查找图像中文档边缘的最佳方法是使用阈值图像。OpenCV 提供了不同的阈值样式,这是由其函数的第 4 个参数决定的。在这个函数中,第一个参数是源图像,这应该是一张灰度图像;第二个参数是用于分类像素值的阈值;第三个参数是 maxVal,这是当像素值超过(有时是低于)阈值时所要给出的值。
下面的代码将能帮助你找到阈值图像,然后确定文档边缘的轮廓,你可以将这些轮廓点与图像边缘进行比较,然后确定文档的边缘。
<p style="box-sizing: border-box;padding: 0.5em;font-size: 14px;color: rgb(169, 183, 198);line-height: 18px;border-radius: 0px;background: rgb(40, 43, 46);display: block;font-family: Consolas, Inconsolata, Courier, monospace;overflow: auto;letter-spacing: 0px;margin-left: 8px;margin-right: 8px;overflow-wrap: normal !important;word-break: normal !important;"><span style="box-sizing: border-box;font-size: inherit;color: rgb(128, 128, 128);line-height: inherit;overflow-wrap: inherit !important;word-break: inherit !important;"># threshold image</span><br style="box-sizing: border-box;font-size: inherit;color: inherit;line-height: inherit;overflow-wrap: inherit !important;word-break: inherit !important;" />ret, thresh = cv2.threshold(imgray, 150, 255, 0)<br style="box-sizing: border-box;font-size: inherit;color: inherit;line-height: inherit;overflow-wrap: inherit !important;word-break: inherit !important;" />cv2.imwrite(<span style="box-sizing: border-box;font-size: inherit;color: rgb(238, 220, 112);line-height: inherit;overflow-wrap: inherit !important;word-break: inherit !important;">'thresh.jpg'</span>, thresh)<br style="box-sizing: border-box;font-size: inherit;color: inherit;line-height: inherit;overflow-wrap: inherit !important;word-break: inherit !important;" /><span style="box-sizing: border-box;font-size: inherit;color: rgb(128, 128, 128);line-height: inherit;overflow-wrap: inherit !important;word-break: inherit !important;"># edge contours</span><br style="box-sizing: border-box;font-size: inherit;color: inherit;line-height: inherit;overflow-wrap: inherit !important;word-break: inherit !important;" />contours, hierarchy = cv2.findContours(thresh, 1, 2)</p>
检测和裁剪/分割文档中的所有词
在有约束的受控环境中进行词检测通常可以使用启发式方法实现,比如利用梯度信息或者这样的事实:文本通常会被分组成段落以及排列成直线的字符。但是,使用启发式方法是存在缺陷的,图像中很多不需要的区域也会被检测为词,所以我们可以使用 OpenCV 的 EAST(Efficient and Accurate Scene Text)检测器。
可以参考 Adrian Rosebrock 写的 EAST 检测器相关文章:https://www.pyimagesearch.com/2018/08/20/opencv-text-detection-east-text-detector/
然后再根据 Tom Hoag 分享的方法对其进行改进:https://medium.com/@tomhoag/opencv-text-detection-548950e3494c
这种方法能以很高的准确度检测出手写文本以及机器打印的文本。检测出图像中的词之后,再将它们裁剪出来并将它们全部保存下来。
预处理词图像
应该怎么样对图像进行预处理?这完全取决于你接下来要做什么。如果想要分类手写的和机器打印的词,需要所有图像都处于灰度模式。为了将图像转换为灰度图像,还需要使用 OpenCV:
<p style="box-sizing: border-box;padding: 0.5em;font-size: 14px;color: rgb(169, 183, 198);line-height: 18px;border-radius: 0px;background: rgb(40, 43, 46);display: block;font-family: Consolas, Inconsolata, Courier, monospace;overflow: auto;letter-spacing: 0px;margin-left: 8px;margin-right: 8px;overflow-wrap: normal !important;word-break: normal !important;"><span style="box-sizing: border-box;font-size: inherit;color: rgb(165, 218, 45);line-height: inherit;overflow-wrap: inherit !important;word-break: inherit !important;">imgray</span> = cv2.cvtColor(img, cv2.COLOR_BGR2GRAY)</p>
这是手写词吗?
这是一个分类问题:确定一张特定图像中的词是「手写词」还是「机打词」。作者浏览了多篇文章和研究论文,发现支持向量机(SVM)是解决这一问题的最佳方案,然后使用了来自 sklearn 软件包的 SVM 分类器来完成这一任务。
对于用于分类的数据集,作者提到了一个很好的手写词图像有标注数据集 IAM:http://www.fki.inf.unibe.ch/databases/iam-handwriting-database
对于机器打印的词图像,作者收集了大约 2000 张词图像。下面是用于预测的特征:

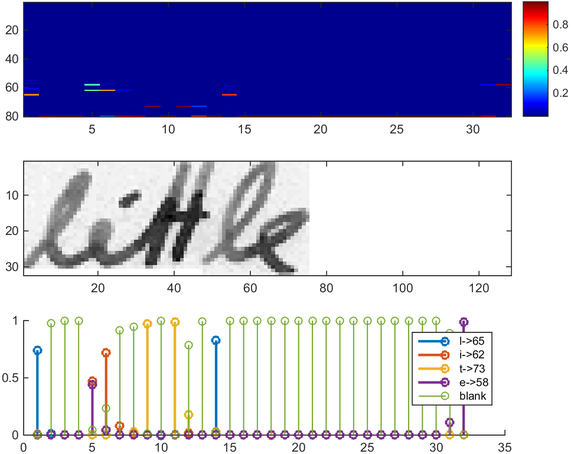
<pre style="max-width: 100%;letter-spacing: 0.544px;box-sizing: border-box !important;overflow-wrap: break-word !important;"><section style="margin-right: 8px;margin-left: 8px;max-width: 100%;white-space: normal;color: rgb(0, 0, 0);font-family: -apple-system-font, system-ui, "Helvetica Neue", "PingFang SC", "Hiragino Sans GB", "Microsoft YaHei UI", "Microsoft YaHei", Arial, sans-serif;letter-spacing: 0.544px;text-align: center;widows: 1;line-height: 1.75em;box-sizing: border-box !important;overflow-wrap: break-word !important;"><strong style="max-width: 100%;box-sizing: border-box !important;overflow-wrap: break-word !important;"><span style="max-width: 100%;letter-spacing: 0.5px;font-size: 14px;box-sizing: border-box !important;overflow-wrap: break-word !important;"><strong style="max-width: 100%;font-size: 16px;letter-spacing: 0.544px;box-sizing: border-box !important;overflow-wrap: break-word !important;"><span style="max-width: 100%;letter-spacing: 0.5px;box-sizing: border-box !important;overflow-wrap: break-word !important;">—</span></strong>完<strong style="max-width: 100%;font-size: 16px;letter-spacing: 0.544px;box-sizing: border-box !important;overflow-wrap: break-word !important;"><span style="max-width: 100%;letter-spacing: 0.5px;font-size: 14px;box-sizing: border-box !important;overflow-wrap: break-word !important;"><strong style="max-width: 100%;font-size: 16px;letter-spacing: 0.544px;box-sizing: border-box !important;overflow-wrap: break-word !important;"><span style="max-width: 100%;letter-spacing: 0.5px;box-sizing: border-box !important;overflow-wrap: break-word !important;">—</span></strong></span></strong></span></strong></section><section style="max-width: 100%;white-space: normal;font-family: -apple-system-font, system-ui, "Helvetica Neue", "PingFang SC", "Hiragino Sans GB", "Microsoft YaHei UI", "Microsoft YaHei", Arial, sans-serif;letter-spacing: 0.544px;text-align: center;widows: 1;box-sizing: border-box !important;overflow-wrap: break-word !important;"><section powered-by="xiumi.us" style="max-width: 100%;box-sizing: border-box !important;overflow-wrap: break-word !important;"><section style="margin-top: 15px;margin-bottom: 25px;max-width: 100%;opacity: 0.8;box-sizing: border-box !important;overflow-wrap: break-word !important;"><section style="max-width: 100%;box-sizing: border-box !important;overflow-wrap: break-word !important;"><section style="max-width: 100%;letter-spacing: 0.544px;box-sizing: border-box !important;overflow-wrap: break-word !important;"><section powered-by="xiumi.us" style="max-width: 100%;box-sizing: border-box !important;overflow-wrap: break-word !important;"><section style="margin-top: 15px;margin-bottom: 25px;max-width: 100%;opacity: 0.8;box-sizing: border-box !important;overflow-wrap: break-word !important;"><section style="max-width: 100%;box-sizing: border-box !important;overflow-wrap: break-word !important;"><section style="margin-right: 8px;margin-bottom: 15px;margin-left: 8px;padding-right: 0em;padding-left: 0em;max-width: 100%;color: rgb(127, 127, 127);font-size: 12px;font-family: sans-serif;line-height: 25.5938px;letter-spacing: 3px;box-sizing: border-box !important;overflow-wrap: break-word !important;"><span style="max-width: 100%;color: rgb(0, 0, 0);box-sizing: border-box !important;overflow-wrap: break-word !important;"><strong style="max-width: 100%;box-sizing: border-box !important;overflow-wrap: break-word !important;"><span style="max-width: 100%;font-size: 16px;font-family: 微软雅黑;caret-color: red;box-sizing: border-box !important;overflow-wrap: break-word !important;">为您推荐</span></strong></span></section><p style="margin-right: 8px;margin-bottom: 5px;margin-left: 8px;padding-right: 0em;padding-left: 0em;max-width: 100%;min-height: 1em;color: rgb(127, 127, 127);font-size: 12px;font-family: sans-serif;line-height: 1.75em;letter-spacing: 0px;box-sizing: border-box !important;overflow-wrap: break-word !important;"><span style="max-width: 100%;box-sizing: border-box !important;overflow-wrap: break-word !important;">“12306”的架构到底有多牛逼?</span></p><p style="margin-right: 8px;margin-bottom: 5px;margin-left: 8px;padding-right: 0em;padding-left: 0em;max-width: 100%;min-height: 1em;color: rgb(127, 127, 127);font-size: 12px;font-family: sans-serif;line-height: 1.75em;letter-spacing: 0px;box-sizing: border-box !important;overflow-wrap: break-word !important;"><span style="max-width: 100%;box-sizing: border-box !important;overflow-wrap: break-word !important;">中国程序员34岁生日当天在美国遭抢笔记本电脑,追击歹徒被拖行后身亡,为什么会发生此类事件?</span></p><p style="margin-right: 8px;margin-bottom: 5px;margin-left: 8px;padding-right: 0em;padding-left: 0em;max-width: 100%;min-height: 1em;color: rgb(127, 127, 127);font-size: 12px;font-family: sans-serif;line-height: 1.75em;letter-spacing: 0px;box-sizing: border-box !important;overflow-wrap: break-word !important;"><span style="max-width: 100%;-webkit-tap-highlight-color: rgba(0, 0, 0, 0);cursor: pointer;font-size: 14px;box-sizing: border-box !important;overflow-wrap: break-word !important;">阿里如何抗住90秒100亿?看这篇你就明白了!</span></p><p style="margin-right: 8px;margin-bottom: 5px;margin-left: 8px;padding-right: 0em;padding-left: 0em;max-width: 100%;min-height: 1em;font-family: sans-serif;line-height: 1.75em;letter-spacing: 0px;box-sizing: border-box !important;overflow-wrap: break-word !important;"><span style="max-width: 100%;color: rgb(87, 107, 149);box-sizing: border-box !important;overflow-wrap: break-word !important;"><span style="max-width: 100%;font-size: 14px;box-sizing: border-box !important;overflow-wrap: break-word !important;">60个Chrome神器插件大收集:助你快速成为老司机,一键分析网站技术栈</span></span><br style="max-width: 100%;box-sizing: border-box !important;overflow-wrap: break-word !important;" /></p><p style="margin-right: 8px;margin-bottom: 5px;margin-left: 8px;padding-right: 0em;padding-left: 0em;max-width: 100%;min-height: 1em;color: rgb(127, 127, 127);font-size: 12px;font-family: sans-serif;line-height: 1.75em;letter-spacing: 0px;box-sizing: border-box !important;overflow-wrap: break-word !important;"><span style="max-width: 100%;-webkit-tap-highlight-color: rgba(0, 0, 0, 0);cursor: pointer;font-size: 14px;box-sizing: border-box !important;overflow-wrap: break-word !important;">深度学习必懂的13种概率分布</span></p></section></section></section></section></section></section></section></section>
本篇文章来源于: 深度学习这件小事
本文为原创文章,版权归知行编程网所有,欢迎分享本文,转载请保留出处!
内容反馈